What Is Artificial Intelligence?
Artificial intelligence (AI) employs algorithms to perform tasks that normally require human intelligence, like playing video games, operating autonomous vehicles and processing language. AI helps people do these activities more smoothly.
AI technology allows humans to make sense of data on an unprecedented scale, increasing productivity and efficiency across industries. But AI also comes with risks, including spreading misinformation or accidentally breaking laws.
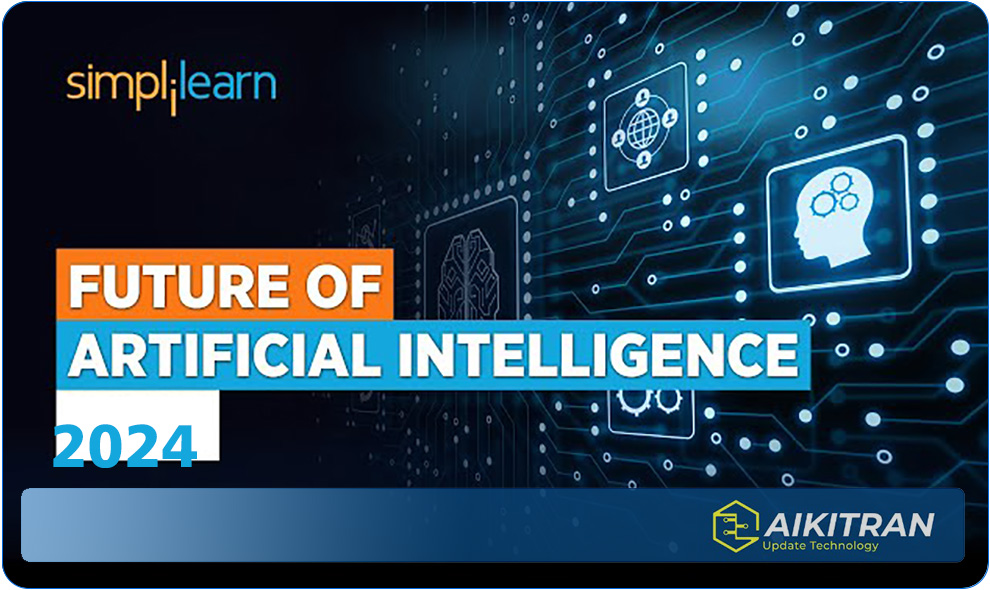
1. Deep Learning: Artificial Intelligence
Deep learning is a type of artificial intelligence (AI) that enables machines to learn from data and adapt accordingly, such as with chess-playing computers or self-driving cars. Deep learning systems collect previous data and use it to improve performance when handling specific tasks; then apply this enhanced performance against similar inputs in future interactions.
Deep Learning is one of the key elements of artificial intelligence (AI) used to help computers comprehend language and perform complex tasks. Deep Learning can be found in speech recognition software such as Siri and Alexa, image and video processing tools such as Google Translate and facial recognition programs such as Clarifai; recommendation algorithms in e-commerce services and streaming services; and robotics automation systems, all of which utilize Deep Learning techniques.
AI can also increase productivity by automating repetitive, high-volume tasks and minimizing human error. Chatbots like Microsoft 365 Copilot offer simple business tasks without human assistance to complete.
However, many fear AI could not only replace jobs but may potentially replace humans altogether. Others worry about an eventuality where intelligent machines evolve so rapidly that they outshout humans in all aspects of life.
This phenomenon, known as artificial superintelligence, or ASI, is still some way off, yet it is still sparking debate on how best to protect human lives while at the same time understanding what makes for intelligence in machines. This includes adaptability and learning capacities, as well as understanding oneself and other entities.
You Must Read…WHAT IS THE CLUBHOUSE APP USE? NEW 1
2. Machine Learning: Artificial Intelligence
Machine learning (ML) is an artificial intelligence field that enables computers to self-learn using data by recognizing patterns within an input of information and applying their findings towards solving future problems.
Artificial intelligence (AI) can be applied in numerous areas, from predictive modeling and natural language processing to computer vision. Predictive models allow businesses to anticipate customer purchasing behavior or determine which patients are at greatest risk of certain diseases; natural language processing helps computers understand human speech for voice recognition software like Amazon Alexa or Apple Siri; and computer vision recognizes images and videos used for things such as self-driving cars or medical imaging.
Personal assistants and AI-powered services that have become ubiquitous with smartphones have made use of Machine Learning in various forms; examples of such include ChatGPT’s use of large language models to respond to any inquiries or comments sent directly to it; Google Translate employs this technology for translation purposes between languages; Amazon and Netflix both employ ML-powered recommendation engines to offer users personalized content based on past searches or purchases;
There is much debate regarding how widespread AI will become in the near future and whether or not it will eventually replace jobs currently performed by humans. No matter its application, however, AI offers great potential benefits, but we must remain mindful that its development could have both positive and negative ramifications on society as a whole.
3. Natural Language Processing
Natural Language Processing (NLP) allows artificial intelligence (AI) to understand and analyze real-world language, both written and spoken, by performing tasks like text and speech analysis, machine translation, and sentiment analysis, often employed in chatbots, search engines, or voice assistants.
NLP techniques include tokenization, which involves breaking words down into their constituent parts, such as “dog” and “barked.” Part-of-speech tagging categorizes each individual word based on its function within a sentence (i.e. nouns, verbs and adjectives). Part of speech tagging further categorizes individual words based on their grammar functions within sentences (such as nouns, verbs, adjectives, etc.). Named entity recognition identifies and categorizes named entities in text data, such as people, brands, locations, dates, or quantities. Sentiment analysis assesses the overall tone of the text, including negative or positive feelings. Natural Language Generation combines databases of information with NLP to generate text that is meaningful and contextually relevant based on the information contained in the text data.
One of the most widely-used types of NLP is large language models (LLM)—computer programs trained on massive amounts of text such as books, articles, and websites to learn patterns in word relationships and recognize patterns among words that help create human-like responses to prompts. LLMs power intelligent assistants like IBM Watson Assistant, Siri, and Amazon Alexa.
NLP can also facilitate intent recognition, which helps identify what a person speaking or writing wants to achieve with specific requests or queries. For instance, when someone requests flight tickets from one airport to another, an AI utilizing NLP technology might return search results with suitable flights from all the airports for each destination and help the customer select their ideal option based on price, availability, and other considerations. You might already be using this type of technology without even realizing it; Grammarly uses machine learning, deep learning and NLP techniques to identify writing errors like misplaced commas found within word processors, texting apps and other forms of written media.
4. Neural Networks
AI may or may not completely replace human workers, but there’s no question that its presence has already altered our work practices. AI applications enable enterprises to produce faster and more accurate results while increasing competitive edge in areas where manual tasks can be automated.
AI and machine learning algorithms have been designed to automate routine tasks, freeing humans to focus on more meaningful work. AI is capable of processing data faster than humans can while uncovering relationships that might otherwise remain hidden; this has the potential to boost productivity while decreasing costs for businesses.
People like yourself interact with Artificial Intelligence every day, from using voice assistants on our smartphones to riding in self-driving cars, but this only scratches the surface when it comes to how AI technology is utilized within businesses.
emergence of artificial intelligence for applications like natural language processing (NLP), computer vision (CV), and robotics is revolutionizing our work lives. NLP allows machines to understand and generate human speech, while CV gives computers the capability of interpreting visual data. Robotics integrates these technologies with mechanical engineering to produce intelligent machines capable of operating autonomously.
Narrow AI employs AI to automate repetitive or rules-based tasks, eliminating human involvement while increasing productivity. Examples of narrow AI include autopilot systems in airplanes, robots that detect cyber threats quickly and respond accordingly, as well as recommendation engines for online retailers that suggest products based on past consumption patterns.
5. Genetic Algorithms
Genetic algorithms (GAs) are heuristic search methods that apply evolutionary and natural selection concepts to solving optimization problems. GAs can quickly find effective solutions for constrained and multi-objective optimization issues; moreover, GAs allow exploration of various regions of search space within relatively short amounts of time and are less prone to becoming stuck in local minima than other techniques.
Initializing a population of candidate solutions and iteratively applying selection, mutation and crossover operators. Each iteration is known as a generation and over time, the algorithm can converge to a near optimal solution.
Genetic representations of candidates can be encoded using binary strings, real-valued numbers or other data types. Genes representing these candidates are then modified, recombined and selected through iterations; replicating natural selection and evolution processes in this process.
Each member of a population is evaluated on performance, and those with high fitness scores are selected as members of the next generation. Their genes then go through selection, mutation and crossover processes once more.
results of iterations that gradually improve quality over time are achieved using genetic algorithms (GAs). A GA can quickly find good solutions in relatively short order, as its iterative approach provides quick solutions. Furthermore, GAs can handle multi-objective problems as well as nonlinear functions whose parameters change with time, making them useful tools in non-stationary (time dependent) and nonlinear functions analysis. DeepMind of Google used one such GA for protein folding technology integrated into consumer products like self-driving cars.
1 thought on “What Is Artificial Intelligence? Best 5 AI”